Generative AI
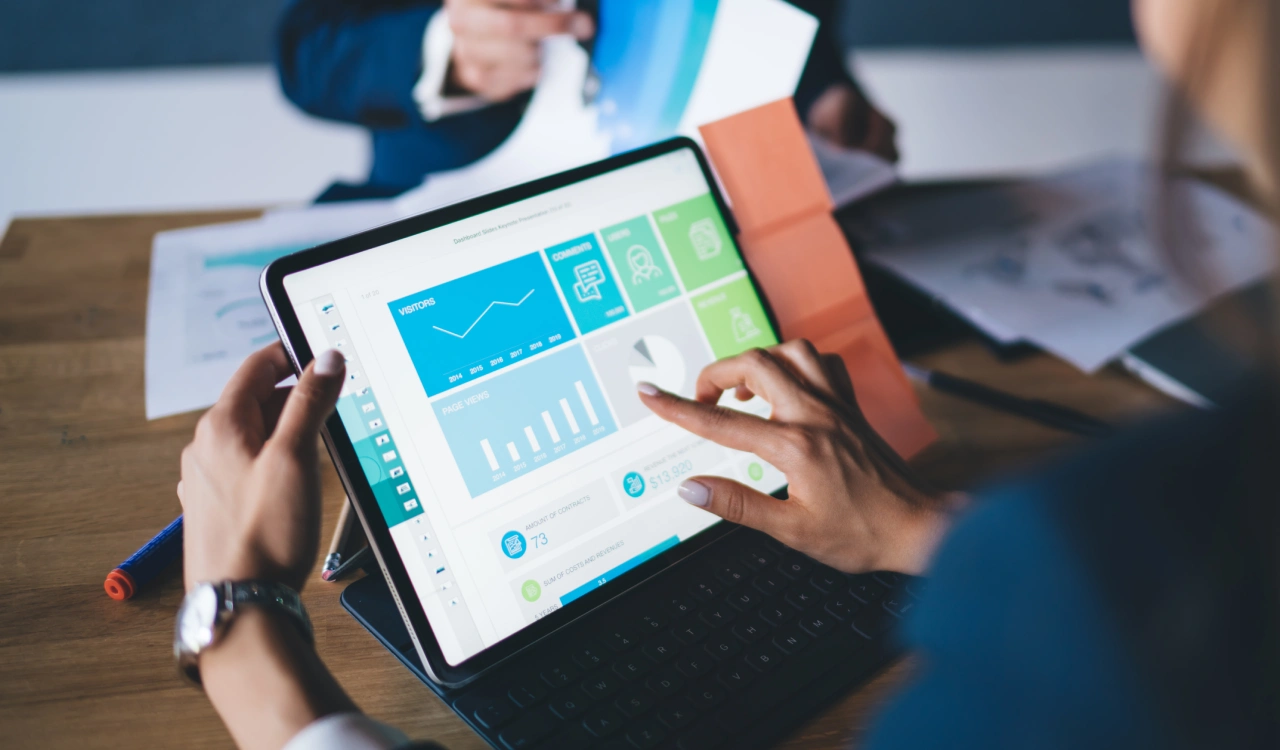
Challenges on the Path of Generative AI Adoption in the Supply Chain
In the world of data management, a new buzzword has emerged – the data lakehouse. It’s a term that is used to describe a modern approach to data architecture that involves a unified platform that provides the advantages of data storage and data processing for various analytics workloads.While Generative AI offers tremendous potential for revolutionizing supply chain operations, its implementation is not without its challenges. In this blog, we will delve into the critical challenges associated with harnessing the power of Generative AI within the supply chain.
Data Availability and Quality
Generative AI models rely on vast volumes of high-quality data to function effectively. Procuring and maintaining reliable data can be a daunting task, especially in supply chain management where data sources are often complex and dynamic. Issues of data collection, cleaning, and integration become critical to ensure the accuracy of Generative AI models.
Model Training and Optimization
Training Generative AI models for supply chain applications can be computationally intensive and time-consuming. It demands significant computational resources and expertise to fine-tune complex models. Selecting the right model architectures and parameters while navigating the balance between complexity and performance is a substantial challenge.
Interpretability and Explainability
In the realm of supply chain decision-making, transparency and understanding the rationale behind generated results are crucial. Generative AI models often require improved interpretability to gain the trust and acceptance of stakeholders.
Real-time Adaptation and Dynamic Environments
Supply chains operate in a constantly changing environment. Generative AI models may struggle to adapt to real-time data, making it essential to incorporate real-time data sources and develop models capable of rapid adaptation.
Ethical and Legal Considerations
Implementing Generative AI in the supply chain raises ethical and legal concerns, particularly when generating synthetic data or content resembling real data. Complying with data privacy regulations, intellectual property rights, and ethical guidelines is vital.
Deployment and Scalability
Integrating Generative AI into existing supply chain systems and processes can be complex. Ensuring seamless integration, scalability, and compatibility with existing infrastructure is a challenging task. Deployment may face obstacles related to compatibility, system disruptions, or additional computational resources.
Addressing these challenges requires a multidisciplinary approach involving expertise in AI, supply chain management, data governance, and legal and ethical considerations. Tackling these hurdles will empower organizations to fully harness the potential of Generative AI, leading to substantial enhancements in operational efficiency and decision-making.
Overcoming the Hurdles for Generative AI in Supply Chain
While Generative AI brings significant promise to the supply chain industry, it is essential to recognize and address the challenges it presents. By navigating these challenges effectively, businesses can utilize Generative AI to its full potential, optimizing supply chain operations and thriving in an increasingly competitive marketplace.