Future Trends in Data Engineering
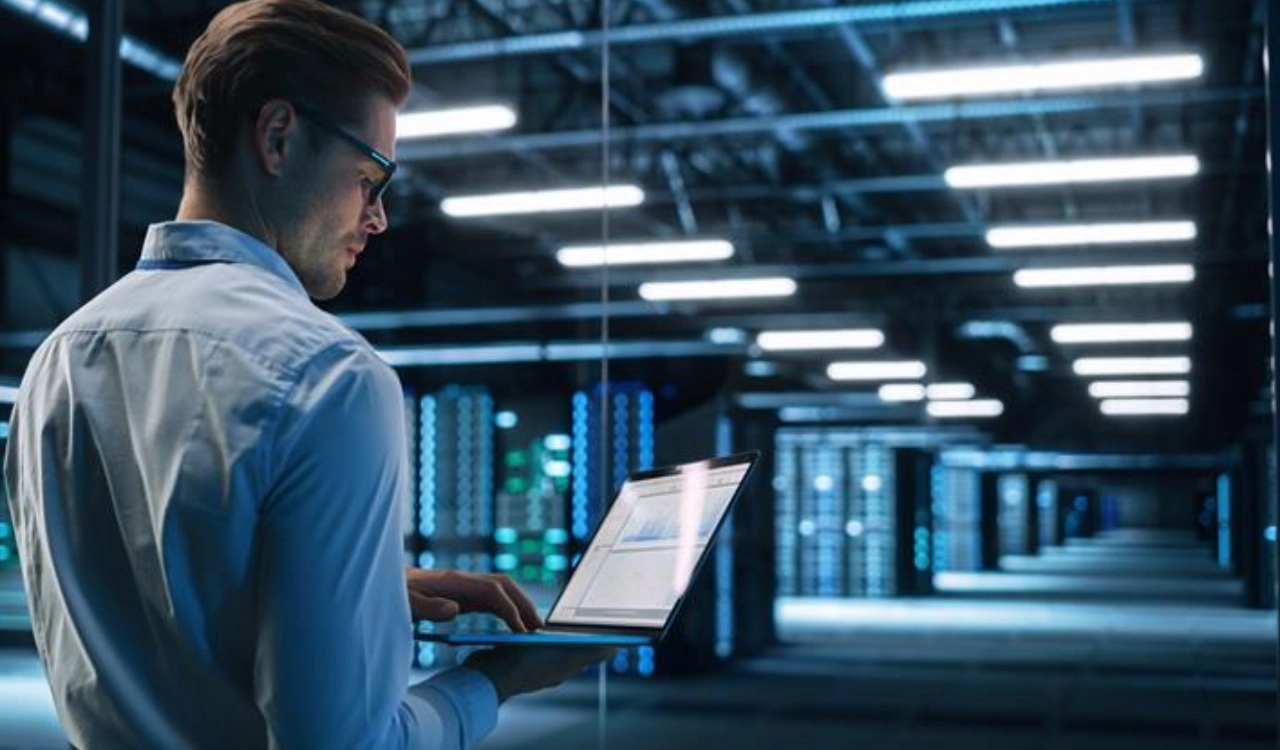
Ever wondered how today’s top companies manage and make sense of the flood of data that keeps growing every second? As businesses grow more dependent on precise, timely data, data engineering has shifted from a specialized function to a business-critical role, it’s become the backbone for managing and optimizing the data that fuels smarter decisions.
In a world where the quality and speed of information can make or break decisions, data engineering has become essential. From creating and optimizing data pipelines to maintaining complex architectures, data engineering ensures that information flows efficiently across systems, driving the quality and flow of information for modern enterprises.
With global data generation projected to surpass 180 zettabytes by 2025, enterprises are focused on how best to capture vast data volumes and convert data into actionable insights. The proof is in the proverbial pudding as in 2022, global spending on cloud infrastructure touched $225 billion. As the sector continues to evolve, new advancements promise to enhance the way organizations collect, process, utilize, and manage data. Let’s dive into some of the most promising engineering trends reshaping 2024, explore the innovations that are redefining data management, storage, and analytics:
Real-time Data Processing
Clarity, visibility, and prompt responses are pertinent for resilient supply chains – this is precisely why real-time data processing dictates a space in an enterprise.
According to research, enhancing visibility in manufacturing and supply chains (55%) is the main business priority for most leaders overall, but this is no longer true for smaller organizations. The growth of real-time data processing has been way more than expected as deriving immediate and scalable insights are necessary to take timely actions. In supply chain, it allows for dynamic management of supply chain, enabling quick responses to disruptions, optimizing inventory levels, and improving delivery times. Technologies such as Apache Kafka and Amazon Kinesis are helping with real-time data streaming and processing.
Use Cases/Trends
- Fraud Detection
Instant identification and prevention of fraud-related activities. - Customer Experience
Providing personalized experiences based on customer behavior. - Operational Efficiency
Monitoring and optimizing processes operationally.
Impact
- Minimizing Data Latency
Reducing latency is essential for real-time AI applications where quick decision-making is critical. Solutions like distributed storage and caching enhance data access speed, boosting model performance in time-sensitive contexts. Software-defined storage (SDS) further balances speed and flexibility across hardware, supporting efficient, rapid scaling. - Enforcing Dynamic Data Quality
Enforcing robust quality rules for accuracy, relevance, and timeliness are vital. Threshold-based alerts and automated rule enforcement can manage inconsistencies across integrated systems (such as ERP and CRM), while dynamic rules allow for flexible adaptation to evolving data demands. - Leveraging AI for Demand Planning
Real-time insights powered by AI improve demand planning by detecting patterns in consumer behavior, economic conditions, and inventory needs. Effective data frameworks that handle real-time updates and streamlined storage are critical to leveraging AI for responsive planning.
Advanced Data Integration
Data Integration still remains a concern as enterprises deal with diverse data sources, sets, and formats. Nearly 60% of enterprises plan to invest in digital technologies, recognizing their potential to boost supply chain efficiency and data analysis. In supply chain, data integration is necessary for digitizing and automating process, and centralizing data across systems for ease of access. This contributes to an agile, resilient, and capable supply chain. In 2024, advanced data integration trends include data virtualization, data fabric, and ML-driven integration.
Use Cases/Trends
- Data Virtualization
Developing a virtual layer to access and query data from different sources without physical movement. - Data Fabric
A unified system that provides easy access and advanced data integration of data across various environments. - ML-driven Integration
Using ML algorithms to enable data mapping, transformation, and integration tasks.
Impact
- Simplified Data Access
Modern data platforms enable more accessible and centralized data access from multiple sources, empowering teams to make faster, data-driven decisions. This fosters collaboration, enhancing visibility and efficiency. - Reduced Complexity
Automated data integration with pre-built connectors reduces the complexity of managing data pipelines, allowing to shift focus from maintenance to innovation. This streamlining accelerates the development of analytics applications. - Enhanced Data Governance
Automated data lineage tracking strengthens compliance and data integrity by documenting data origin, usage, and transformations. This robust governance framework supports regulatory compliance and ensures high data quality.
AI and ML Integration
As AI and ML continue to forge through the supply chain ecosystem, 50 % of enterprises will surprisingly take the bait and invest in applications that support artificial intelligence and advanced analytics capabilities.
Integrating AI and ML into data engineering workflows has become prevalent and more importantly relevant in the data engineering field. It involves embedding ML models directly into data pipelines to enrich data processing related work. In supply chain, these integrations have the capacity to enhance supplier relationships, provide better demand forecasting, and improve inventory forecasting while providing a clear view of inventory-based management.
Use Cases/Trends
- Data Cleaning
Using integrated AI into workflows to detect and correct data anomalies and errors. - Predictive Maintenance
Monitoring and analyzing data to predict and ensure there are no operational failures. - Personalization
Real-time customization of data and recommendations based on the data generated by the system.
Impact
- Enhancing Data Quality through Cleaning
Integrating AI into data cleaning processes significantly improves data quality by automating the identification and correction of anomalies. This results in enhanced accuracy of insights derived from data, enabling enterprises to make more informed decisions. High-quality data is crucial for remaining agile to customer needs. - Proactive Management with Predictive Maintenance
Leveraging AI for predictive maintenance ensures no potential operational failures. This proactive strategy minimizes downtime, reduces maintenance costs, and extends the lifespan of equipment. By preventing unexpected disruptions, businesses can maintain reliability and operational efficiency. - Elevating Customer Engagement through Personalization
Using AI significantly enhances the customer experience by providing relevant and timely suggestions. This personalized approach fosters customer loyalty and increases engagement, ultimately driving higher conversion rates. Tailoring offerings based on user interactions enables enterprises to relate more closely to consumer preferences.
Cloud Native Data Engineering
Since the early 2010s, cloud native data engineering has become the norm as enterprises migrate their data workloads to the cloud. Although the legacy systems are quite ancient, the current refined Cloud platforms are scalable, flexible, and cost-effective. Little did we know that AI and cloud computing are expected to grow at a CAGR of approximately 11.1%, potentially reaching $85.3 billion by 2033.
With potential billions, cloud native data engineering is touted to ease the business. Specifically, in supply chain, Cloud systems assist with sales, match demand with available supply chain sources, and receive status updates for order fulfilment so that an enterprise can improve planning and resource allocation.
Use Cases/Trends
- Serverless Computing
Reducing dependency on building and managing infrastructure to focus on building sophisticated data engineering applications. - Containerization
Using software applications for consistent deployment and orchestration of data services without delays. It ensures security as the apps are bundled to run on any operating system faster without damaging any software or hardware. - Data Lakes and Warehouses
Leveraging cloud-native data storage solutions like Amazon and Azure.
Impact
- Scalability
The ability to adjust resources based on demand is essential for managing fluctuating workloads effectively. This allows optimization of their data infrastructure, ensuring sufficient resources during peak periods while minimizing costs during lulls. This adaptability is vital for supporting growth. - Flexibility
Access to a diverse range of tools and services for various data engineering tasks enhances an enterprise’s ability to customize solutions according to specific needs. This flexibility fosters innovation. By leveraging various applications, teams can effectively address emerging challenges and optimize their data processes. - Cost Efficiency
Some Cloud models significantly lower upfront costs, making advanced data engineering solutions more accessible. This cost efficiency allows organizations to invest in robust data systems without incurring large capital expenditures. By aligning costs with actual usage, teams can optimize budgets and reallocate resources to other critical areas.
Data Governance and Compliance
It’s not entirely unforeseen that good quality data smoothens a wrecked system. But according to Gartner, the impact is huge – 70% of organizations will rigorously track data quality levels via metrics, improving it by 60% to significantly reduce operational risks and costs.
Data regulation and compliance have been stringent due to multiple fraud and sensitive data leaks. Robust data governance and compliance frameworks are necessary. Within supply chain, relevant, accurate, and reliable data is vital to establish trust and motivate it’s adoption by users of our system applications. The current trends in this practice include automated governance and compliance mechanisms.
Use Cases/Trends
- Data Lineage
Tracking the legitimate source and transformation of data from the start to finish in its entire lifecycle. - Access-based Controls
Implementing role-based access control (RBAC) to secure sensitive data and ensure there is a multiple step authentication system in place. - Data Audit Sequence
Keeping comprehensive logs of data from its origin for auditing and compliance purposes.
Impact
- Credibility
Effective compliance strategies help manage data responsibly, enhancing customer loyalty and trust. By implementing transparent governance practices, enterprises build confidence among clients and regulators. This trust is essential in today’s data-driven economy, where consumers demand accountability. - Lower Risk
Prioritizing data governance not only mitigates risks but also distinguishes enterprises in the marketplace, attracting customers who value security and integrity. Implementing strong security measures and compliance frameworks minimizes the likelihood of data breaches and associated costs. - Regulatory Compliance
Following data protection regulations such as GDPR, CCPA, and HIPAA are essential for enterprises that handle personal data. It not only avoids legal penalties but also enhances an enterprise’s reputation among consumers who are increasingly aware of their privacy rights.
Other than these key trends in data engineering that affects supply chain, a few other trends and predictions that impact data engineering are:
- Rise of Data Ops
The combination of data engineering and operations – Data Ops, is gaining significant momentum. There has been automation of data pipeline processes that can rise collaboration within teams and departments. This trend will most likely see widespread adoption, emphasizing skill bridging in continuous integration and delivery for data. - Enhanced Data Visualization and Self-Serving Analytics
To make data more accessible tools such as Tableau, Power BI, and Looker will gain momentum. It will allow non-tech users to explore and visualize data, creating a culture of data-driven decisions within an organization. - Data Mesh
A decentralized way forward between organizational design and data architecture, where data ownership is shared with teams that can use the data and create their own product or application with said data. It will help in creating an agile data management policy that can help achieve supply chain goals easily. - Data Automation and AI
Applications will be developed to ensure processes such as data integration, quality control, and ETL tasks can be managed and run by AI single-handedly without human intervention. It will reduce the manual effort, speed by data pipelines, and reduce errors freeing up the data engineering tasks to work on meaningful aspects of data engineering. - Data Cataloging and Observability
A till now narrowly utilized feature within the data engineering world, this technology will provide greater visibility into the data landscape and its operational metrics that can improve its overall efficiency. It will ensure high availability and reliability of inventory if used well within supply chain systems in an enterprise.
2024 and the upcoming year in data engineering will present both significant challenges but exciting growth prospects as well. The increasing use of real-time analytics, cloud architecture, integration of AI and ML are set to change how we ingest, manage, and utilize data. By closely monitoring these future trends and developing the right form of data engineering applications, supply chain optimization will be effortless and data-driven in the future for all our customers.
Want another detailed observation on what the future of data engineering holds? Let us know in the comments section.
*Syren Cloud is a keen observer of future trends in data engineering that improves and makes supply chains agile. All views are based on our applications and real-time interactions with our clients.
Fill out the form below to connect with our supply chain experts and explore our solutions. Schedule a free demo!