The Evolution of Generative AI
- Introduction
- Traditional AI Vs. Generative AI in Supply Chain Management
- Applications of Generative AI in Supply Chain Management
- Limitations of Generative AI in Supply Chain Management
- Challenges in Leveraging Generative AI in
Supply Chain Management
- Data Availability and Quality of Generative Ai in Supply Chain Management
- Model Training and Optimization
- Investment Challenges
- Interpretability and Explainability of Generative AI in Supply Chain Management
- Real-time Adaptation of Generative AI in Supply Chain Management
- Ethical Considerations of Leveraging Generative AI in Supply Chain Management
- Conclusion
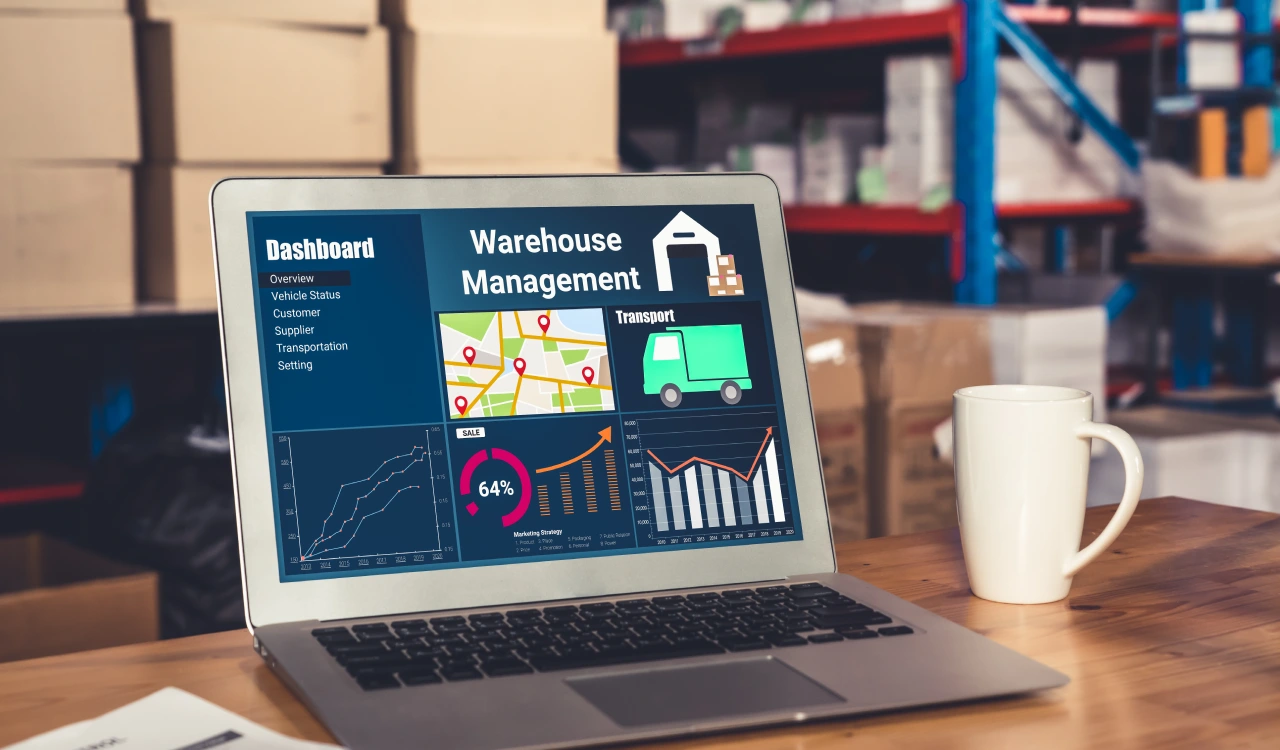
Introduction
Supply chains are the backbone of global commerce, but they’re also complex and vulnerable to disruptions. By learning from vast amounts of data, generative AI can not only help automate tasks but also creatively solve problems and propose innovative solutions. From design and manufacturing to fulfillment and service, generative AI has the potential to transform every stage of the supply chain, making it faster, more efficient, and more resilient.
Read On: From Algorithms to Aisles, Check Out How AI is Revolutionizing Supply Chains
Traditional AI Vs. Generative AI in Supply Chain Management
Generative AI and traditional AI represent distinct approaches within the broader field of artificial intelligence. While they share common goals, they have fundamental differences in terms of their underlying techniques and applications. In this blog, we’ll explore these differences to help you understand which approach is better suited for specific tasks.
Category | Traditional AI | Generative AI |
---|---|---|
Focus | Traditional AI excels at tasks with clear rules and goals. It works by following established instructions, making it ideal for situations where predictable outcomes are desired. This approach is perfect for tasks like data analysis based on well-defined rules or automating specific processes with clear parameters. | Generative AI primarily concentrates on creating new and original content, such as text, images, audio, and synthetic data. It excels in creative fields and is valued for its capacity to autonomously generate diverse types of outputs. |
Techniques | Traditional AI utilizes well-defined models and frameworks. It leverages techniques like convolutional neural networks, recurrent neural networks, and reinforcement learning to process data and achieve specific goals. | Generative AI leverages advanced neural network architectures, such as transformers, Generative Adversarial Networks (GANs), and Variational Autoencoders (VAEs), to produce novel content. |
Data Usage | Traditional AI models rely heavily on historical data to make predictions and decisions. They use this data to identify patterns and develop algorithms that can be applied to solve specific problems. | Generative AI models use data in a fundamentally different way. Instead of relying solely on historical data, these models analyze the underlying data distributions to generate new, creative content that mimics the patterns in the training data. |
Interaction and Collaboration | Traditional AI models rely more on pre-defined rules and algorithms, with less direct human interaction during the training process. The development of these models often involves extensive data cleaning, feature engineering, and model tuning by human experts. | Generative AI models often receive more direct human input during the training process, learning from information and feedback provided by humans. This interaction can help the models develop a better understanding of the problem domain and generate more relevant and useful outputs. |
Adaptability to Ambiguity | Unlike generative AI, traditional AI shines in well-defined tasks. It excels when rules are clear and outcomes predictable, thanks to its structured approach to data. However, this makes it less suited for handling ambiguity or constantly evolving data. | Generative AI thrives in uncertainty. It excels at tasks with unclear data or open interpretations, generating creative solutions where traditional, rule-based AI struggles. This makes it perfect for exploring uncharted territories. |
Read On: Check Out How Generative AI is Bringing Transformative Opportunities in CPG Industries
Applications of Generative AI in Supply Chain Management
Generative AI holds significant promise for revolutionizing supply chain management by offering advanced and smart capabilities across various key areas. Let us delve into the various applications of generative AI in supply chain management processes:
Gen AI-powered Procurement
Generative AI in supply chain can streamline supplier evaluation processes by analyzing diverse factors, for instance, generative AI helps ingest data from various sources, including structured data (e.g., supplier quotes, performance metrics) and unstructured data (e.g., news articles, social media posts, customer reviews). This enables more informed decision-making and optimized contract negotiations.
Manufacturing and Production
Firstly, generative AI accelerates innovation by enabling rapid generation and testing of countless ideas. Secondly, it reduces costs by minimizing wasted resources and production delays through early identification of optimal designs. This accelerates production processes and enhances operational efficiency.
Inventory Optimization With Gen AI
Managing inventory levels is a balancing act. Generative AI can help predict optimal stock levels by considering various variables, preventing overstocking and reducing excess inventory. It identifies efficient distribution and storage strategies. The system uses real-time insights to prevent overstock and oversupply which in turn reduces inventory holding costs. minimizing costs and enhancing responsiveness.
Logistics Optimization With Gen AI in Supply Chain Management
Generative AI algorithms can help businesses to optimize trade networks and transportation routes operations. It factors in everything from traffic to weather patterns to recommend the most efficient routes, saving costs and time. It can also dynamically adjust routes for disruptions and explore multimodal transportation options. This not only optimizes delivery but also fosters a more resilient and sustainable supply chain.
Returns Management and Sustaibaility Tracking
Generative AI helps businesses in tracking and managing carbon emissions, particularly focusing on carbon footprint and emission sources. This capability empowers enterprises to abide by their sustainability initiatives and align with environmental goals. Take control of your carbon footprint with Syren. Our Sustainability Tracker leverages data analytics to pinpoint emission sources and guide the development of customized emission reduction plans.
Personalization With Generative AI in Supply Chain Management
Through sophisticated data analysis, generative AI can help deliver personalized product recommendations and tailored customer experiences. By leveraging customer feedback and preferences, businesses can enhance customer satisfaction and loyalty.
Read Further: 7 Ways AI is Revolutionizing Customer Experiences
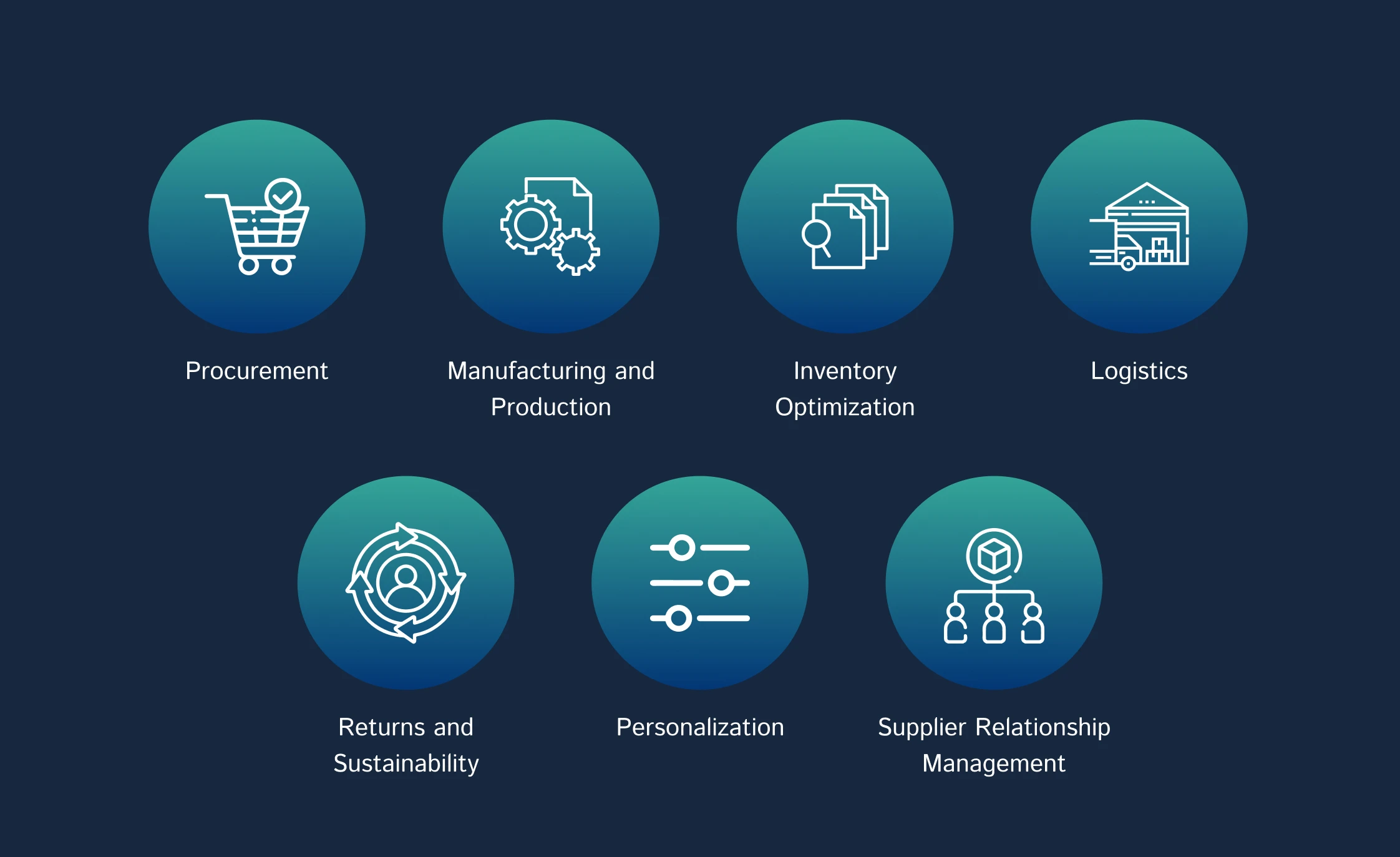
Supplier Selection and Relationship Management
Choosing reliable suppliers and managing relationships is critical. Generative AI helps businesses analyze a wide range of data to assist in supplier selection, enhancing supply chain resilience. It also helps in managing relationships, providing insights to address challenges and improve collaborations.
Read Further: Learn More About the Ways AI Enables Ethical Sourcing Practices
Limitations of Generative AI in Supply Chain Management
Data Quality
Generative AI heavily relies on data quality for accurate results. Ensuring data accuracy and reliability is crucial to avoid biased or inaccurate outcomes that could impact decision-making processes.
Ethical Considerations
AI-generated decisions must align with ethical and legal standards. Enterprises need to monitor AI algorithms regularly to prevent biased or unfair outcomes, ensuring compliance with regulations and ethical guidelines.
Investment Challenges
Integrating generative AI into existing supply chain systems may require significant effort and investment. Enterprises must carefully plan and execute the integration process to ensure seamless operation and compatibility with existing systems.
Human Expertise
While generative AI offers efficiency and automation, human expertise remains essential for effective procurement, risk management, and supplier relationship management. Over-reliance on AI models without human oversight can lead to potential breakdowns and disruptions in operations.
While generative AI presents numerous benefits for supply chain management, enterprises must address limitations related to data quality, ethical considerations, integration challenges, human expertise, and compliance to fully leverage its potential and ensure effective implementation in supply chain operations.
Challenges in Leveraging Generative AI in Supply Chain Management
While generative AI offers tremendous potential for revolutionizing supply chain operations, its implementation is not without its challenges.
Data Availability and Quality of Generative Ai in Supply Chain Management
Generative AI models rely on vast volumes of high-quality data to function effectively. Procuring and maintaining reliable data can be a daunting task, especially in supply chain management where data sources are often complex and dynamic. Issues of data collection, cleaning, and integration become critical to ensure the accuracy of Generative AI models.
Model Training and Optimization
Training Generative AI models for supply chain applications can be computationally intensive and time-consuming. It demands significant computational resources and expertise to fine-tune complex models. Selecting the right model architectures and parameters while navigating the balance between complexity and performance is a substantial challenge.
Interpretability and Explainability of Generative AI in Supply Chain Management
In the process of supply chain decision-making, transparency and understanding the rationale behind generated results are crucial. Generative AI models often require improved interpretability to gain the trust and acceptance of stakeholders.
Real-time Adaptation of Generative AI in Supply Chain Management
Supply chains operate in a constantly changing environment. Generative AI models may struggle to adapt to real-time data, making it essential to incorporate real-time data sources and develop models capable of rapid adaptation.
Ethical Considerations of Leveraging Generative AI in Supply Chain Management
Implementing generative AI in the supply chain raises ethical and legal concerns, particularly when generating synthetic data or content resembling real data. Complying with data privacy regulations, intellectual property rights, and ethical guidelines is vital.
While generative AI presents numerous benefits for supply chain management, enterprises must address limitations related to data quality, ethical considerations, integration challenges, human expertise, and compliance to fully leverage its potential and ensure effective implementation in supply chain operations.
Addressing these challenges requires a multidisciplinary approach involving expertise in AI, supply chain management, data governance, and legal and ethical considerations. Tackling these hurdles will empower enterprises to fully harness the potential of generative AI, leading to substantial enhancements in operational efficiency and decision-making.
Generative AI presents a transformative opportunity for supply chain management, offering enhanced forecasting, optimized inventory management, real-time decision support, and more. However, to completely leverage its potential, enterprises must address challenges related to data quality, ethical considerations, integration complexities, and human expertise. By overcoming these hurdles and ensuring responsible implementation, businesses can unlock the true power of generative AI and drive significant improvements in their supply chain operations.
Click Here to Learn How: Generative AI Helps Accelerate Processes in the Retail Sector
Fill out the form below to connect with our supply chain experts & schedule a free demo!